From Patches to Pictures (PaQ-2-PiQ): Mapping the Perceptual Space of Picture Quality
Blind or no-reference (NR) perceptual picture quality prediction is a difficult, unsolved problem of great consequence to the social and streaming media industries that impacts billions of viewers daily. Unfortunately, popular NR prediction models perform poorly on real-world distorted pictures. To advance progress on this problem, we introduce the largest (by far) subjective picture quality database, containing about 40000 real-world distorted pictures and 120000 patches, on which we collected about 4M human judgments of picture quality. Using these picture and patch quality labels, we built deep region-based architectures that learn to produce state-of-the-art global picture quality predictions as well as useful local picture quality maps. Our innovations include picture quality prediction architectures that produce global-to-local inferences as well as local-to-global inferences (via feedback).
LIVE-FB Large-Scale Social Picture Quality Database
The LIVE-FB Large-Scale Social Picture Quality Database includes 39,810 images and 119,430 patches extracted from them, on which we collected about 4M quality scores in total from 7,865 unique subjects.
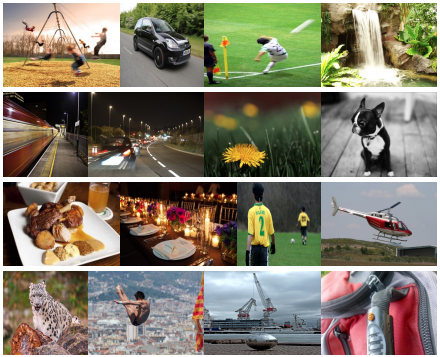
Examples
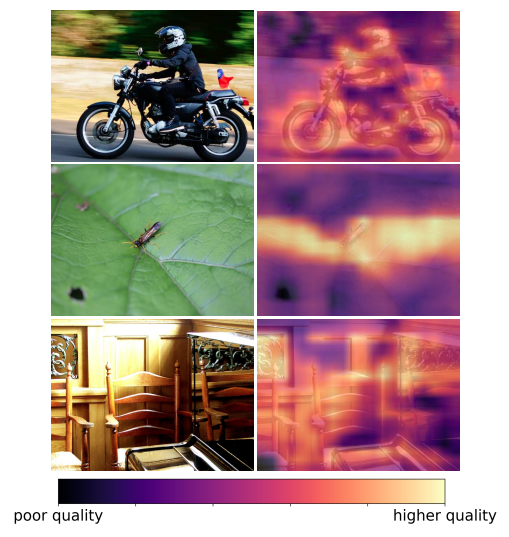
References
- D. Ghadiyaram and A. C. Bovik. Massive online crowdsourced study of subjective and objective picture quality. IEEE Transactions on Image Processing, vol. 25, no. 1, pp. 372-387, Jan 2016. 2, 3, 4, 5, 8, 12
- Z. Ying, M. Mandal, D. Ghadiyaram, and A. Bovik, "PatchVQ:'patching up' the video quality problem, arXiv preprint arXiv:2011.13544, 2020.
- D. Ghadiyaram and A. C. Bovik. Perceptual quality prediction on authentically distorted images using a bag of features approach. Journal of Vision, vol. 17, no. 1, art. 32, pp. 1-25, January 2017. 2
- H. R. Sheikh, M. F. Sabir, and A. C. Bovik. A statistical evaluation of recent full reference image quality assessment algorithms. IEEE Transactions on Image Processing, vol. 15, no. 11, pp. 3440-3451, Nov 2006. 2, 3, 4
- H. Lin, V. Hosu, and D. Saupe. Koniq-10K: Towards an ecologically valid and large-scale IQA database. arXiv preprint arXiv:1803.08489, March 2018. 2, 3, 4, 5, 8, 12