End-to-End Statistical Learning, with or without Labels
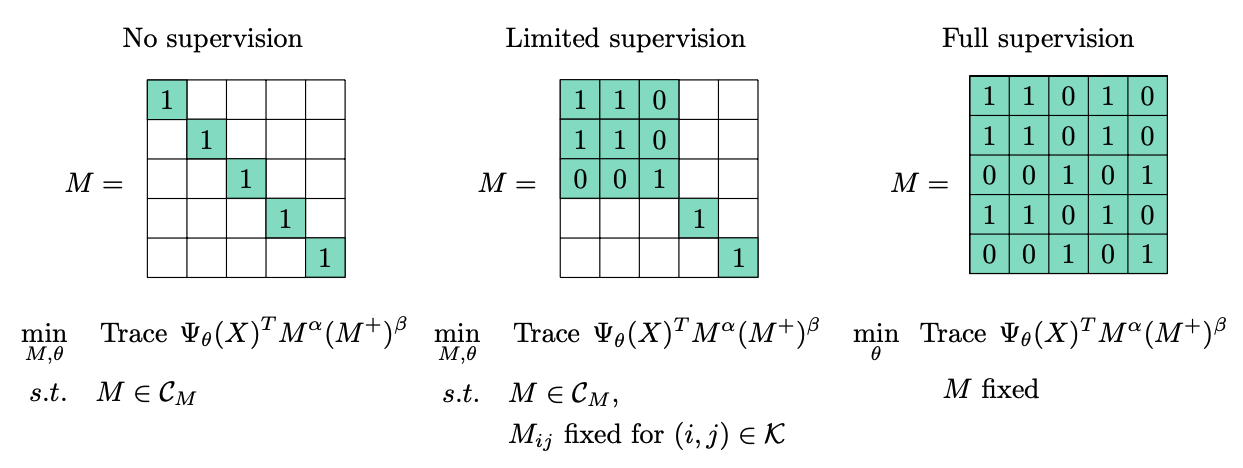
In [1], we present a discriminative clustering approach in which the feature representation can be learned from data and moreover leverage labeled data. Representation learning can give a similarity-based clustering method the ability to automatically adapt to an underlying, yet hidden geometric structure. The proposed approach is an extension of a discriminative clustering method called DIFFRAC. We show how to augment DIFFRAC with a representation learning capability, using a gradient-based stochastic training algorithm and an entropy-regularized optimal transport algorithm. The resulting method is evaluated on several real datasets when varying the ratio of labeled data to unlabeled data and thereby interpolating between the fully unsupervised regime and the fully supervised regime. The experimental results suggest that the proposed method can learn powerful feature representations even in the fully unsupervised regime and can leverage even small amounts of labeled data to improve the feature representation and to obtain better clusterings of complex datasets.
Results
Our approach is called XSDC for X-Supervised Discriminative clustering where X can be ‘un’, ‘semi’ or ‘’ highlighting that all regimes of supervision (as one varies the ratio of labeled data to unlabeled data) are covered by the approach. With XSDC, the representation, i.e., the deep network, is trained on both labeled and unlabeled data. We compare XSDC to a network whose parameters are learned only from the labeled data and a network whose parameters are set randomly. The test accuracies on several datasets (MNSIT, Gisette, MAGIC, CIFAR10) with a given deep network are plotted as the proportion of labeled to unlabeled data vary.
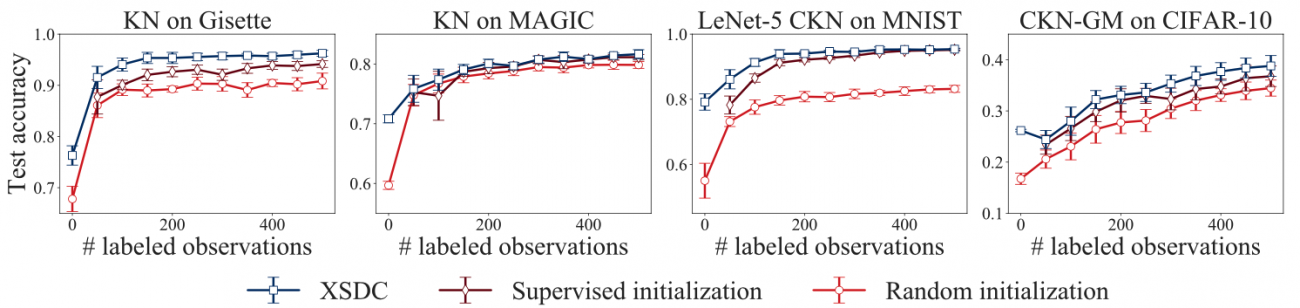