Events
IFML Seminar
IFML Seminar: 02/28/25 - Bridging Generative and Discriminative Learning with Contrastive Mutual Information Machine
-The University of Texas at Austin
Gates Dell Complex (GDC 6.302)
2317 Speedway
Austin, TX 78712
United States
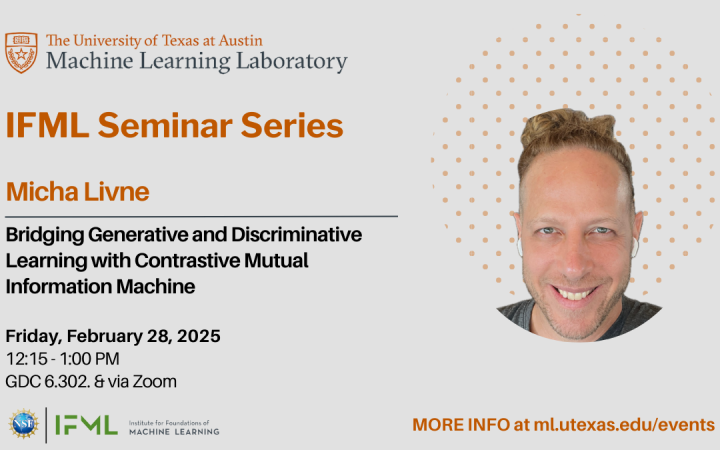
Abstract: Learning representations that generalize across diverse downstream tasks is a fundamental challenge in machine learning. Contrastive learning, self-supervised masking, and denoising autoencoders have emerged as powerful paradigms, yet a unified approach that effectively supports both generative and discriminative tasks remains elusive.
In this talk, we introduce contrastive Mutual Information Machine (cMIM), a novel learning framework that enhances representation utility by integrating contrastive learning with the Mutual Information Machine (MIM)—a symmetric variational autoencoder that maximizes mutual information between observations and latent representations while encouraging clustered structure. While MIM’s generative properties are promising, its representations were found to underperform in discriminative tasks compared to state-of-the-art (SOTA) models. cMIM directly addresses this limitation.
Our contributions are twofold:
1. We propose a contrastive extension to MIM that learns discriminative representations without requiring data augmentation and is robust to batch size variations.
2. We introduce a generic method for extracting informative embeddings from encoder-decoder models, significantly improving discriminative task performance without additional training.
Beyond representation learning, we will discuss how statistical principles in cMIM enable greater control in generative tasks, such as molecular design, while also improving embeddings for discriminative applications. Our results demonstrate that cMIM achieves a unique balance between generative expressiveness and discriminative power, offering a principled approach to unified representation learning.
In this talk, we introduce contrastive Mutual Information Machine (cMIM), a novel learning framework that enhances representation utility by integrating contrastive learning with the Mutual Information Machine (MIM)—a symmetric variational autoencoder that maximizes mutual information between observations and latent representations while encouraging clustered structure. While MIM’s generative properties are promising, its representations were found to underperform in discriminative tasks compared to state-of-the-art (SOTA) models. cMIM directly addresses this limitation.
Our contributions are twofold:
1. We propose a contrastive extension to MIM that learns discriminative representations without requiring data augmentation and is robust to batch size variations.
2. We introduce a generic method for extracting informative embeddings from encoder-decoder models, significantly improving discriminative task performance without additional training.
Beyond representation learning, we will discuss how statistical principles in cMIM enable greater control in generative tasks, such as molecular design, while also improving embeddings for discriminative applications. Our results demonstrate that cMIM achieves a unique balance between generative expressiveness and discriminative power, offering a principled approach to unified representation learning.
Bio: Micha Livne is a Senior Research Scientist at NVIDIA, specializing in machine learning, generative models, and representation learning. His work spans latent variable models, Bayesian inference, and self-supervised learning, with applications in bioinformatics and cheminformatics. He has contributed extensively to NeMo and BioNeMo, developing foundational encoder-decoder architectures and advancing generative AI for molecular and protein modeling.
Micha holds a PhD in Computer Science from the University of Toronto, where he worked under Professor David Fleet, focusing on the Mutual Information Machine (MIM) framework. Prior to NVIDIA, he held research roles at Disney Research, Adobe, and Mitsubishi Electric Research Labs. His work explores unified generative and discriminative learning, aiming to enhance the effectiveness of AI across diverse applications.
Event RegistrationMicha holds a PhD in Computer Science from the University of Toronto, where he worked under Professor David Fleet, focusing on the Mutual Information Machine (MIM) framework. Prior to NVIDIA, he held research roles at Disney Research, Adobe, and Mitsubishi Electric Research Labs. His work explores unified generative and discriminative learning, aiming to enhance the effectiveness of AI across diverse applications.