Events
Responsible Machine Learning's Causal Turn: Promises and Pitfalls
Zachary Lipton, PhD, Assistant Professor, Carnegie Mellon University
-The University of Texas at Austin
Gates Dell Complex (GDC 6.302)
United States
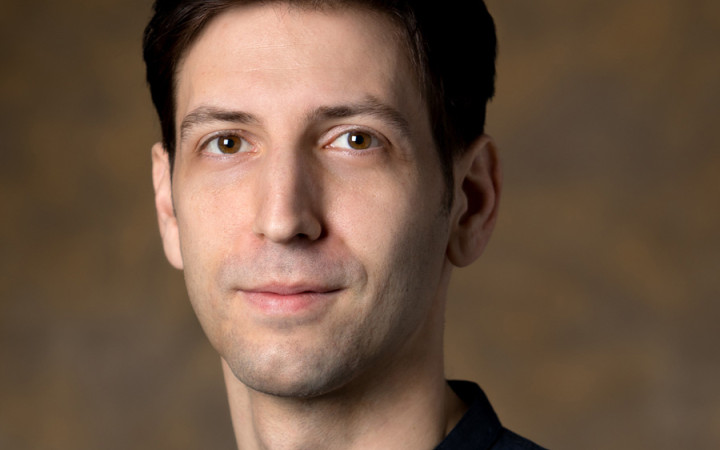
Abstract: With widespread excitement about the capability of machine learning systems, this technology has been instrumented to influence an ever-greater sphere of societal systems, often in contexts where what is expected of the systems goes far beyond the narrow tasks on which their performance was certified. Areas where our requirements of systems exceed their capabilities include (i) robustness and adaptivity to changes in the environment, (ii) compliance with notions of justice and non-discrimination, and (iii) providing actionable insights to decision-makers and decision subjects. In all cases, research has been stymied by confusion over how to conceptualize the critical problems in technical terms. And in each area, causality has emerged as a language for expressing our concerns, offering a philosophically coherent formulation of our problems but exposing new obstacles, such as an increasing reliance on stylized models and a sensitivity to assumptions that are unverifiable and (likely) unmet. This talk will introduce a few recent works, providing vignettes of reliable ML’s causal turn in the areas of distribution shift, fairness, and transparency research.
Bio: Dr. Zachary Lipton is an Assistant Professor of Machine Learning and Operations Research at Carnegie Mellon University (CMU). He holds appointments in the Machine Learning Department in the School of Computer Science (primary), Tepper school of Business (joint), Heinz School of Public Policy (courtesy) and Societal Computing (courtesy). Dr. Lipton’s research spans core machine learning methods and theory, their applications in healthcare and natural language processing, and critical concerns, both about the mode of inquiry itself, and the impact of the technology it produces on social systems. He is director of the Approximately Correct Machine Learning Intelligence Lab.
Event Registration