Events
IFML Seminar
Fantastic Sparse Masks and Where to Find Them
Shiwei Liu, PhD
-The University of Texas at Austin
Gates Dell Complex (GDC 6.302)
United States
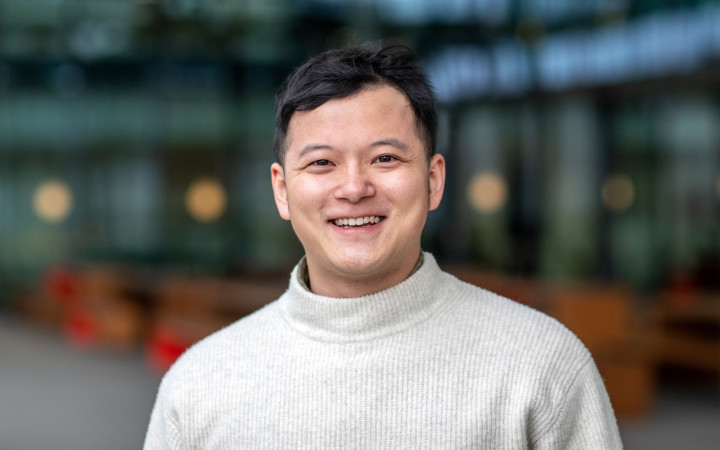
Abstract: Sparsity has widely shown its versatility in model compression, robustness improvement, and overfitting mitigation by selectively masking out a portion of parameters. However, traditional methods to obtain such masks usually involve pre-training a dense model. As powerful foundation models become prevailing, the cost of the pre-training step can be prohibitive. In this talk, I will present our recent work on efficient methods to obtain such fantastic masks by training sparse neural networks from scratch, without the need for any dense pre-training steps.
Bio: Shiwei Liu joined IFML in Fall 2022 as a postdoctoral fellow in the VITA group, under the supervision of Dr. Atlas Wang. His research interests include (1) designing efficient (sparse) neural networks and training recipes in pursuit of efficient training and inference; and (2) studying the behavior of deep learning from a scientific perspective, by conducting empirical experiments in practice. Shiwei obtained his Ph.D. degree at the Eindhoven University of Technology (TU/e), the Netherlands, with the Distinguished Dissertation Award, under the supervision of Prof. Mykola Pechenizkiy and Dr. Decebal Constantin Mocanu. He has recently co-received the best paper award from the inaugural Learning on Graphs (LoG) Conference 2022 (as the senior author).
Event Registration