Events
Workshop
-
Mathematics of Deep Learning Workshop
The University of Texas at Austin Machine Learning Lab
Gates Dell Complex (GDC 6.302)
Austin, TX 78712
United States
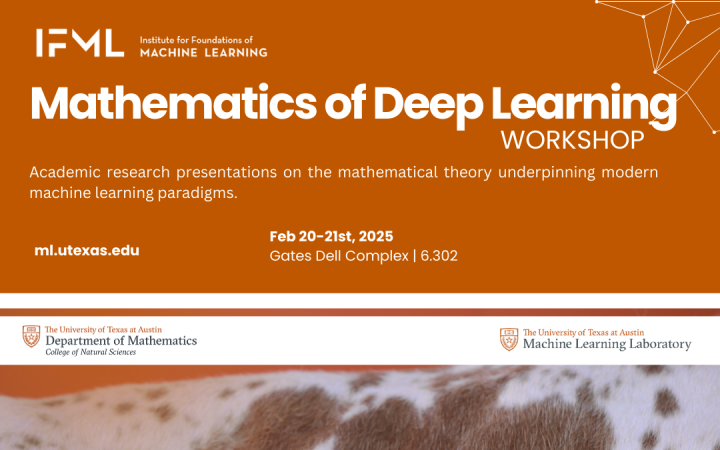
This workshop will be held in the Gates Dell Complex, room 6.302.
Capacity is limited to 70; kindly use the linked Eventbrite to register for this free event.
Agenda:
Feb 20, 2025
8:30-9:00am Coffee and Breakfast
9:00-9:45am Joan Bruna, Professor of Computer Science, Data Science and Mathematics at the Courant Institute and Center for Data Science, New York University
- Title: Posterior Sampling with Denoising Oracles via Tilted Transport
- Abstract: Score-based diffusion models learn a denoising oracle (or score) from datasets that provides an efficient sampling scheme going beyond typical isoperimetric assumptions. From a Bayesian perspective, they offer a realistic modeling of data priors, used for solving inverse problems through posterior sampling. Although many heuristic methods have been developed recently for this purpose, they lack the quantitative guarantees needed in many scientific applications. In this talk, focusing on linear inverse problems, we introduce the tilted transport technique. It leverages the quadratic structure of the log-likelihood in combination with the prior denoising oracle to transform the original posterior sampling problem into a new `boosted' posterior that is provably easier to sample from, along the so-called Polchinski flow [Beaudineau, Bauerschmidt, Dagallier]. We quantify the conditions under which this boosted posterior is strongly log-concave, highlighting the dependencies on the condition number of the measurement operator and the signal-to-noise ratio. The resulting posterior sampling scheme is shown to reach the computational threshold for sampling Ising models with a direct analysis, and is further validated on high-dimensional Gaussian mixture models and scalar field phi-4 models.
9:55-10:40am Vardan Papyan, Assistant Professor, Department of Mathematics and Department of Computer Science, University of Toronto
- Talk: Block Coupling and its Correlation with Generalization in LLMs and ResNets
- Abstract: In this talk, we dive into the internal workings of both Large Language Models and ResNets by tracing input trajectories through model layers and analyzing Jacobian matrices. We uncover a striking phenomenon—block coupling—where the top singular vectors of these Jacobians synchronize across inputs or depth as training progresses. Interestingly, this coupling correlates with better generalization performance. Our findings shed light on the intricate interactions between input representations and suggest new pathways for understanding training dynamics, model generalization, and Neural Collapse.
10:40-11:00am Coffee Break
11:00-11:45am Thomas Chen, Professor of Mathematics, University of Texas at Austin
- Talk: Explicit construction of global minimizers and the interpretability problem in Deep Learning
- Abstract: In this talk, we present some recent results aimed at the rigorous mathematical understanding of how and why supervised learning works. We point out genericness conditions related to reachability of zero loss minimization and underparametrized versus overparametrized Deep Learning (DL) networks. For underparametrized DL networks, we explicitly construct global, zero loss cost minimizers for sufficiently clustered data. In addition, we derive effective equations governing the cumulative biases and weights, and show that gradient descent corresponds to a dynamical process in the input layer, whereby clusters of data are progressively reduced in complexity ("truncated") at an exponential rate that increases with the number of data points that have already been truncated. For overparametrized DL networks, we prove that the gradient descent flow is homotopy equivalent to a geometrically adapted flow that induces a (constrained) Euclidean gradient flow in output space. If a certain rank condition holds, the latter is, upon reparametrization of the time variable, equivalent to simple linear interpolation. This in turn implies zero loss minimization and the phenomenon known as “Neural Collapse”. A majority of this work is joint with Patricia Munoz Ewald (UT Austin).
12:00-12:45pm Jonathan Siegel, Assistant Professor, Mathematics Department at Texas A&M University.
- Talk: Continuous Invariant Neural Networks via Weighted Frame Averaging
- Abstract: In many practical applications of machine learning, especially to scientific disciplines like physics, chemistry, or biology, the ground truth satisfies some known symmetries. Mathematically, this corresponds to invariance or equivariance of the prediction function with respect to a certain group of symmetries, typically the rotation or permutation groups. As a simple example, the chemical properties of a molecule are invariant to rotations. We will discuss the problem of building symmetries into deep neural network architectures. One way of doing this is to canonicalize the input to the network, for example to rotate the molecule into a standard position before passing it into the network. We will show that a major deficiency of this approach is that in most cases of interest it cannot preserve continuity of the neural network. To rectify this, we introduce a generalization called weighted frame averaging and construct efficient weighted frames for the actions of permutations and rotations on point clouds.
Break for Individual Lunch
2:30-4:00pm - Three graduate student talks, 25 mins each
Elzbieta Polak (Mathematics, UT Austin)
- Title: Optimization of Shallow Neural Networks
- Abstract: In this talk I will go over results regarding optimization of shallow neural networks with polynomial activations. The function space for these models can be identified with a set of symmetric tensors with bounded rank. The optimization problem in this setting can be viewed as a low-rank tensor approximation with respect to a non-standard inner product, induced by the data distribution. I will introduce the notion of the discriminant suitable in this context. Based on joint work with Yossi Arjevani, Joan Bruna, Joe Kileel, and Matthew Trager.
Patrícia Ewald (Mathematics, UT Austin)
- Title: Geometric interpretations of ReLU neural networks via truncation maps
- Abstract: We present explicit constructions of feedforward ReLU neural networks that classify data with zero loss using truncation maps, providing a geometric interpretation for the roles of depth and width. Building on joint work with Thomas Chen (UT Austin), we extend our analysis from clustered and sequentially linearly separable data to more complex scenarios, such as concentric data.
–
Yifan Zhang (Oden Institute for Computational Engineering and Sciences, UT Austin)
- Title: Covering number of real algebraic varieties and beyond: improved bound and applications
- Abstract: We prove an upper bound on the covering number of real algebraic varieties, images of polynomial maps and semialgebraic sets. The bound remarkably improves the best known general result by Yomdin and Comte (2004), and its proof is much more straightforward. As a consequence, our result gives new bounds on the volume of the tubular neighborhood of the image of a polynomial map and a semialgebraic set, where results for varieties by Lotz (2015) and Basu, Lerario (2022) are not directly applicable. In this talk I will discuss these results and illustrate its applications to low rank tensor decomposition and statistical learning theory.
Feb 21, 2025
8:30-9:00am Coffee and Breakfast
9:00-9:45am - Richard Tsai, Professor Department of Mathematics and Oden Institute for Computational Engineering and Sciences, The University of Texas at Austin
- Talk: Challenges of Learning from Lower-Dimensional Data Manifolds and Potential Remedies
- Abstract: In this talk, we explore the challenges of learning a function from data that is concentrated around a lower-dimensional manifold. In such cases, the sensitivity of the learned function is influenced by the degree of concentration, which can impact inference stability. To address these issues, appropriate regularization techniques are essential. We will discuss various regularization strategies tailored to different applications and their effectiveness in mitigating these challenges.
9:55-10:40am - Nhat Ho, Assistant Professor Department of Statistics and Data Sciences, The University of Texas at Austin
- Talk: Foundation of Mixture of Experts in Large-Scale Machine Learning Models
- Abstract: Mixtures of experts (MoEs), a class of statistical machine learning models that combine multiple models, known as experts, to form more complex and accurate models, have been combined into deep learning architectures to improve the ability of these architectures and AI models to capture the heterogeneity of the data and to scale up these architectures wit out increasing the computational cost. In mixtures of experts, each expert specializes in a different aspect of the data, which is then combined with a gating function to produce the final output. Therefore, parameter and expert estimates play a crucial role by enabling statisticians and data scientists to articulate and make sense of the diverse patterns present in the data. However, the statistical behaviors of parameters and experts in a mixture of experts have remained unsolved, which is due to the complex interaction between gating function and expert parameters. In the first part of the talk, we investigate the performance of the least squares estimators (LSE) under a deterministic MoEs model where the data are sampled according to a regression model, a setting that has remained largely unexplored. We establish a condition called strong identifiability to characterize the convergence behavior of various types of expert functions. We demonstrate that the rates for estimating strongly identifiable experts, namely the widely used feed-forward networks with activation functions sigmoid(·) and tanh(·), are substantially faster than those of polynomial experts, which we show to exhibit a surprising slow estimation rate. In the second part of the talk, we show that the i sights from theories shed light into improving important practical applications, including enhancing the performance of Transformer model with a novel self-attention mechanism, efficiently finetuning large-scale AI models for downstream tasks, and effectively scaling up massive AI models with several billion parameters.
10:40-11:00am - Coffee Break
11:00-12:00pm - Yoav Wald, Faculty Fellow at the Center for Data Science, New York University
- Talk: Theoretical and empirical insights on learning under spurious correlations and shortcuts
- Abstract: Predictive models often exploit spurious correlations, leading to unreliable performance under distribution shift. In this talk, we explore causally motivated approaches to mitigating spurious correlations and improving model robustness. We begin with a simple model for understanding spurious correlations and their impact on generalization. Then we discuss three aspects of developing robust models: (i) Considerations in training overparameterized models that maintain invariance to shifts in spurious correlations, (ii) Approaches to dealing with scenarios where annotations of spurious features are unavailable, (iii) Leveraging large language models and causal estimation techniques to enhance robustness in large-scale, real-world medical text classification tasks.
12:15-1:00pm - Eli Grigsby, Professor of Mathematics, Boston College
- Talk: Local complexity measures in modern parameterized function classes for supervised learning
- Abstract: The parameter space for any fixed architecture of neural networks serves as a proxy during training for the associated class of functions - but how faithful is this representation? For any fixed feedforward ReLU network architecture, it is well-known that many different parameter settings can determine the same function. It is less well-known that the degree of this redundancy is inhomogeneous across parameter space. I'll discuss two locally-applicable complexity measures for ReLU network classes and what we know about the relationship between them: (1) the local functional dimension, and (2) a local version of VC dimension called persistent pseudodimension. The former is easy to compute on finite batches of points, the latter should give local bounds on the generalization gap. I'll speculate about how this circle of ideas might help guide our understanding of the double descent phenomenon. All of the work described in this talk is joint with Kathryn Lindsey. Some portions are also joint with Rob Meyerhoff, David Rolnick, and Chenxi Wu.
1:00-2:00 - Faculty Lunch (GDC 4.202)
2:30-4:00pm - Three graduate student talks, 25 mins each
Zehua Lai (Mathematics, UT Austin)
- Title: The expressive strength of ReLU transformers
- Abstract: In this talk, we will explain two works on the algebraic structure of ReLU transformers. We first explain the closed connection of ReLU-transformers and piecewise continuous functions. Viewed in this manner, this mysterious but critical component of a transformer becomes a natural development of an old notion deeply entrenched in classical approximation theory. We will further give quantitative bounds on the parameters of transformers.
Liangchen (Lewis) Liu (Mathematics, UT Austin)
- Title: Sloppiness in DNN training and multirate gradient descent
- Abstract: Sloppiness refers to a system's varying sensitivity to changes in different parameter sets. This characteristic is evident in fields like physics, biology, and notably in the training of deep neural networks (DNNs), as revealed through Hessian spectrum analysis. Our research suggests that this sloppiness partly stems from the data distribution's spectrum. Building on top of this understanding, we introduce a gradient descent algorithm that employs both small and large learning rates, tailored to the system’s multi-scale eigenvalue structure, to enhance the optimization efficiency.
Medha Agarwal (Department of Statistics, University of Washington)
- Title: Heat Flows through Pretrained Transformers
- Abstract: Self-attention matrices lie at the heart of transformer-based architecture. The transformation of input data under consecutive self-attention layers can be interpreted as a system of interacting particles evolving over time. Building off the inspiring work of Sander et al. (2022), we present a theoretical analysis of this evolution process, its limiting dynamics, and its convergence to a heat flow, within a framework of gradient flows in the space of probability measures. We also propose a novel discretization scheme for gradient flows that involves successfully computing Schrödinger bridges with equal marginals. This is different from both the forward/geodesic approximation and the backward/Jordan-Kinderlehrer-Otto (JKO) approximations. We prove the uniform convergence of this discretization scheme to the gradient flow in the 2-Wasserstein metric. We present several numerical illustrations of our theoretical results. This is joint work with Garrett Mulcahy, Soumik Pal, and Zaid Harchaoui.