Events
IFML Seminar
IFML Seminar: 12/13/24 - Safe and Informative Imaging via Conformal Prediction and Generative Models
Phil Schniter, Professor, Electrical and Computer Engineering The Ohio State University
-The University of Texas at Austin
Gates Dell Complex (GDC 6.302)
2317 Speedway
Austin, TX 78712
United States
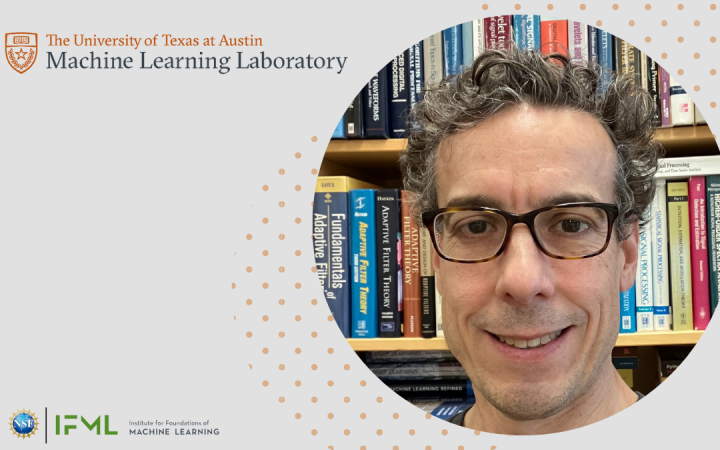
Abstract: Deep neural networks (DNNs) have become popular tools to solve ill-posed image recovery problems, such as those associated with accelerated magnetic resonance imaging (MRI), limited angle computed tomography (CT), phase retrieval, and so on. But modern DNNs sometimes hallucinate, i.e., generate visually pleasing recoveries that differ in important ways from the true image. Thus, there's a strong need to quantify the accuracy of a given recovery, especially in safety-critical applications like medical imaging.
We describe recent work from our group that employs techniques from conformal prediction to rigorously bound the quality of an image recovery relative to the true image without knowing the true image. The notion of "quality" is generic and includes, e.g., PSNR, SSIM, or perceptually motivated metrics like LPIPS. We also consider task-based imaging, where the final goal is to extract quantitative information about the true image from ill-posed measurements. An example would be extracting the probability that the image belongs to some class (e.g., healthy vs unhealthy patients). In this case, conformal prediction can provide rigorous probabilistic bounds on the true task output. In both cases, it's essential that the conformal bounds are adapt to the measurements on hand, so that additional measurements can be taken if needed. We provide this capability by leveraging, and improving on, recent work on approximate posterior sampling methods for imaging inverse problems. Along that latter thrust, we describe our recent work on (unsupervised) diffusion methods for inverse problems that achieve state-of-the-art accuracy over a range of computational budgets, as well as (supervised) conditional GANs that achieve similar accuracy with a single neural function evaluation (NFE).
Bio: Phil Schniter is a Professor in the ECE department at The Ohio State University in Columbus, OH. He earned BS and MS degrees in EE from UIUC, a Ph.D. degree in EE from Cornell University, and is a Fellow of the IEEE. His current research focuses on machine learning for signal and image processing.
Event Registration