IFML Podcast
Research at the forefront of AI and Machine Learning
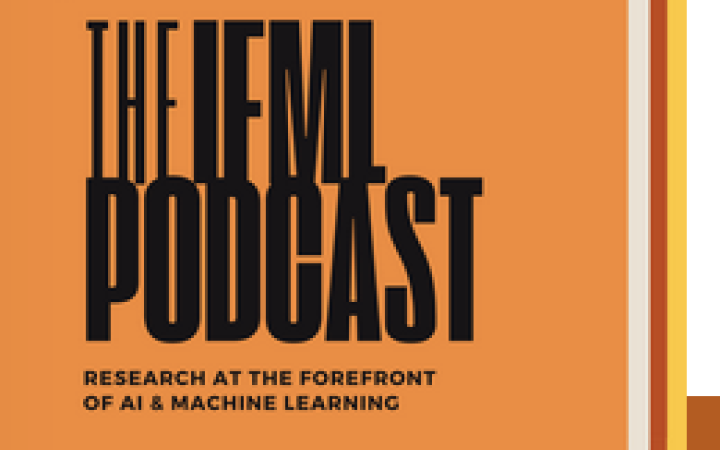
Generative AI has made remarkable strides in the last few years, producing text that's indistinguishable from human prose and incredibly photorealistic images and videos. Automatically measuring how close the generated data distribution is to the target distribution is central to diagnosing existing models and developing better ones. In this first episode of the IFML Podcast, Adam Klivans and Alex Dimakis sit down with the research team behind MAUVE, an automated metric that measures the gap between neural text and human text.
Listen to the EP01: MAUVE on Apple, Spotify, or the IFML Podcast website.
Hosts: Adam Klivans and Alex Dimakis
Guests: Krishna Pillutla, John Thickstun, Zaid Harchaoui

Krishna Pillutla is an assistant professor in the Department of Computer Science & Engineering at IIT Madras. Previously, he was a visiting researcher at Google Research in Mountain View, CA with the Federated Learning team. Krishna received his PhD from the Paul G. Allen School of Computer Science & Engineering at the University of Washington, under the advisement of Zaid Harchaoui and Sham Kakade. Prior to that, he worked with Nina Balcan for his Master’s degree at Carnegie Mellon University and received an undergraduate degree from IIT Bombay. Krishna’s research has been recognized by an Outstanding Paper Award at NeurIPS 2021, a J.P. Morgan Ph.D. fellowship (2019), and two Honourable Mentions for the American Statistical Association (ASA) Student Paper Award.
John Thickstun is a postdoctoral researcher at Stanford University, advised by Percy Liang. Previously, he completed a PhD at the University of Washington, advised by Sham M. Kakade and Zaid Harchaoui. John is interested in developing principled methods that advance the capabilities and controllability of generative models. His work has been featured in media outlets including TechCrunch and the Times of London, recognized by outstanding-paper awards at NeurIPS and ACL, and supported by an NSF Graduate Fellowship and a Qualcomm Innovation Fellowship.
Zaid Harchaoui is a professor at the University of Washington in Seattle, in the Department of Statistics and in the Allen School of Computer Science and Engineering, and a Senior Data Science Fellow in the eScience Institute. He is an action editor at the Journal of Machine Learning Research. He is a principal investigator and a cofounder of IFML, the NSF-AI Institute on Foundations of Machine Learning, and of IFDS, the NSF-TRIPODS institute on foundations of data science. He obtained the doctoral degree from Telecom Paris - Institut Polytechnique de Paris, for his research performed at CNRS - the French National Institute for Fundamental Research. He previously held appointments at the Courant Institute of Mathematical Sciences at New York University, and at INRIA - the French National Institute for Research in Digital Science and Technology. His research has been recognized by several awards, including a NeurIPS outstanding paper award and a CIFAR fellowship.