Events
IFML Seminar
Finite-Sum Coupled Compositional Optimization: Theories and Practical Applications
Tianbao Yang, Associate Professor, CSE, Texas A&M University
-The University of Texas at Austin
GDC 6.302
United States
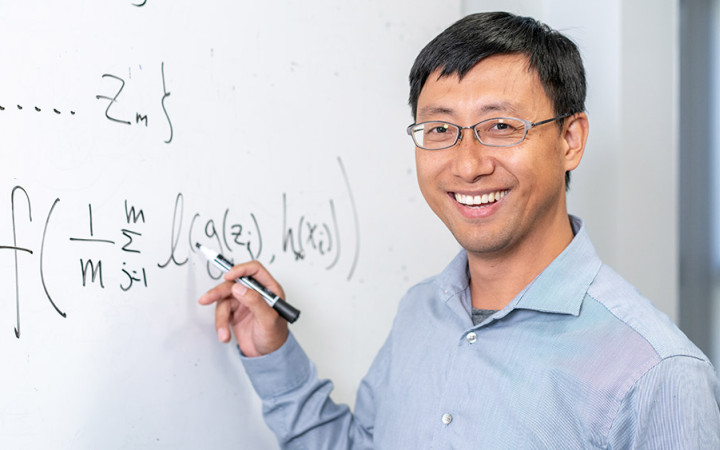
Abstract: During this presentation, I will delve into an innovative class of optimization problems called finite-sum coupled compositional optimization (FCCO). First, I will explore the rationale behind employing FCCO as a framework for addressing issues that arise when optimizing non-decomposable losses in the context of machine learning (ML) and artificial intelligence (AI). Next, I will delve into the algorithms and their associated convergence guarantees for different subclasses of FCCO problems. Finally, I will present our research on the practical uses of FCCO. This includes achievements such as maximizing Average Precision for deep learning that help secure victory in the MIT AI Cures Challenge and addressing the large-batch size problem in contrastive self-supervised learning through global contrastive loss minimization, among other noteworthy applications.
Speaker Bio: Tianbao Yang is an Associate Professor at CSE department of Texas A&M University, where he directs the lab of Optimization for Machine learning and AI (OptMAI Lab). His research interests center around optimization, big data, machine learning and responsible AI. Before joining TAMU, he was an assistant professor and then tenured Dean's Excellence associate professor at the Computer Science Department of the University of Iowa from 2014 to 2022. He received my Ph.D. degree in Computer Science from Michigan State University in 2012, and received my Bachelor degree from University of Science and Technology of China in 2007. He worked in Silicon Valley as Machine Learning Researcher for two years at GE Research and NEC Labs. He received the Best Student Paper Award of COLT in 2012, and the NSF Career Award in 2019. He is the founder of the LibAUC library.