How New Machine Learning Techniques Could Improve MRI Scans
Jon Tamir's Research Highlighted on Amazon Science
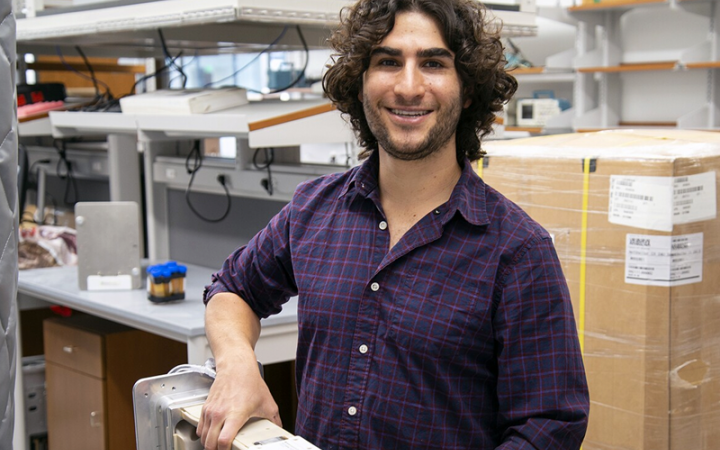
For many patients, time moves at a glacial pace during a magnetic resonance imaging (MRI) scan. Those who have had one know the challenge of holding impossibly still inside a buzzing, knocking scanner for anywhere from several minutes to more than an hour.
Jonathan (Jon) Tamir is developing machine learning methods to shorten exam times and extract more data from this essential — but often uncomfortable — imaging process.
MRI machines use the body's response to strong magnetic fields and radiofrequency waves to produce pictures of our insides, helping to detect disease and monitor treatments. Just like any image, an MRI scan begins with raw data. Tamir, who is an assistant professor of electrical and computer engineering at the University of Texas at Austin, wants to improve how that data is acquired and derive better images faster. In 2020, he earned an Amazon Machine Learning Research Award and funding from the IFML to support the work. Read the full story on Amazon Science.