Turbocharging Protein Engineering with AI
Biotech advances from UT’s new Deep Proteins group are changing the game with help from artificial intelligence.
Marc Airhart, Anita Shiva
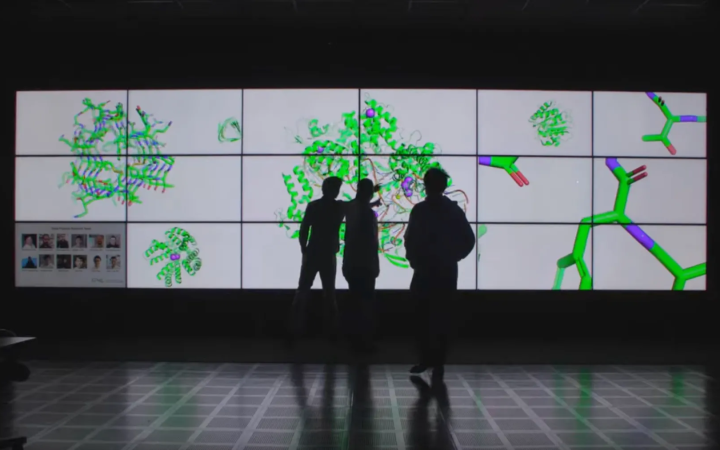
Working as a chemist in Houston, Danny Diaz spent a lot of time plodding his way through crosstown traffic, pondering how to speed up his research.
“I realized that my impact in the short term would be limited to the amount of chemistry experiments I could do with my hands,” he recalled.
It occurred to him that with software engineering and data analytics, he could run virtual experiments any time, even while he slept or was traveling. And the results could help him bypass some of the trial and error that’s common in the lab.
When he took this mindset with him into a chemistry graduate program at The University of Texas at Austin, he arrived at a starting line of sorts along with other researchers around the world who were just beginning to develop ambitious new approaches to designing proteins for a host of useful applications ranging from therapeutics to agrochemicals to food additives to enzymes that break down plastic waste. Among the most promising applications for protein engineering are therapeutics to treat diseases such as cancer, autoimmune disorders, rare metabolic disorders, Alzheimer’s, infectious diseases and more. Because the traditional way of developing proteins into therapeutics is slow, expensive and riddled with failure, it was a field primed for disruption.
In 2020, when Diaz was a second-year Ph.D. student, Google’s DeepMind team released the second version of AlphaFold, a powerful artificial intelligence tool for predicting what three-dimensional shape any given protein would take, based on its sequence of amino acids. Predicting these protein shapes, called structures, had been a grand challenge in biology for decades and, although the model’s predictions weren’t perfect, further refinements have brought it so close that the problem is now considered more or less solved.
The key to AlphaFold’s success is a new paradigm for building AI models, called the transformer architecture, which is also the foundation of generative AI tools like ChatGPT. While most folks know it as a way to generate useful text, researchers in biology and chemistry quickly realized that transformer models could also be applied to designing protein-based biotechnologies. AlphaFold has enabled many of Diaz’s research projects, where he uses it to train AI models with protein structures where experimental data doesn’t exist.
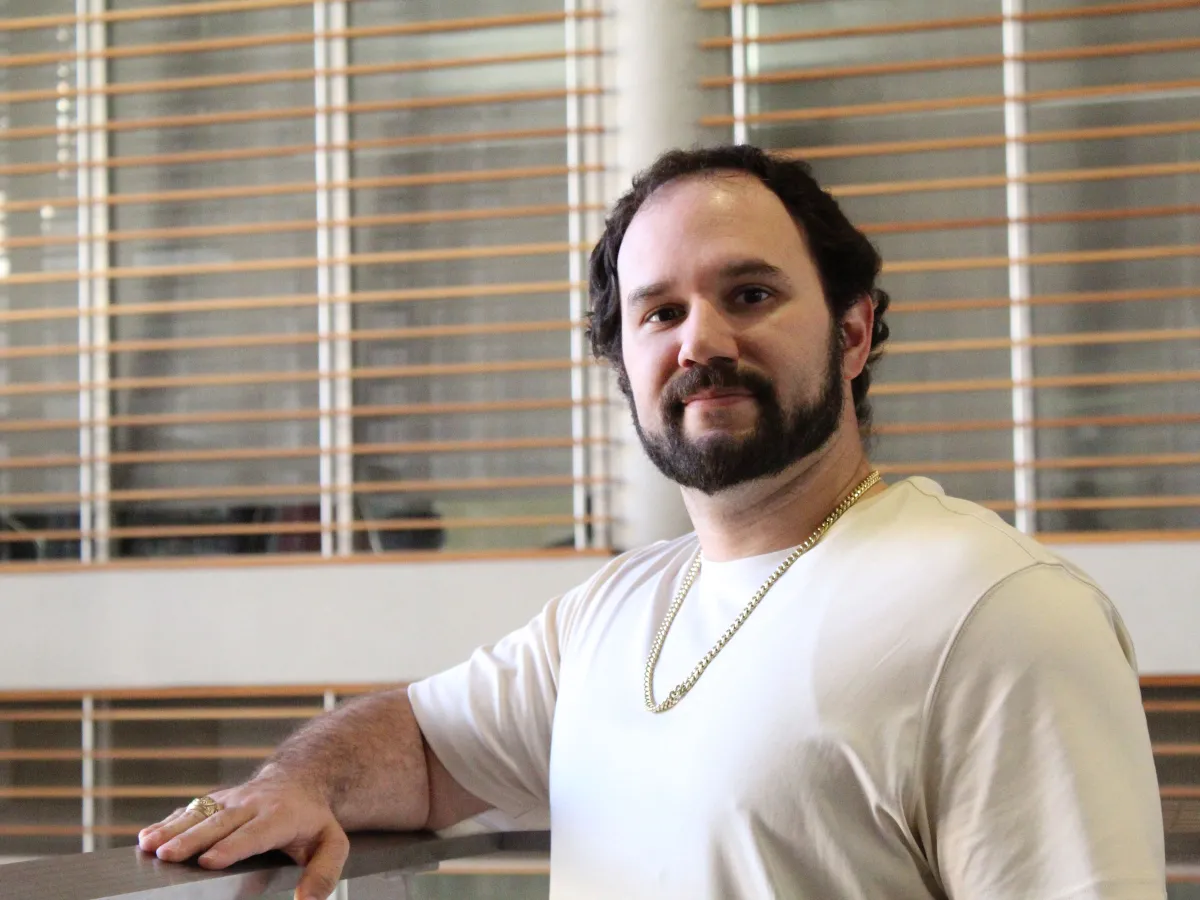
Planning for Proteins
You might recall from high school biology that proteins are made up of amino acids strung together like beads on a necklace. The exact order of the amino acids, or the protein sequence, determines the 3D shape it will take and how it will function. However, there are more potential variations of protein sequences than stars in the universe, with most of these variations being unstable or non-functional. This makes testing them in the lab to find improved, highly functional proteins extremely arduous.
To make a protein useful for applications in health and medicine often requires introducing variations, via genetic engineering, so that a protein can be used in a vaccine, as a cancer drug or to biomanufacture goods. Identifying mutations that optimize proteins is difficult, also, because it traditionally relies on scientists leveraging scarce data in the scientific literature related to any specific protein to guide their intuition.
Enter artificial intelligence. The dream is that AI models can learn from a wide range of data sources and then guide researchers to better designs faster. Professor Andrew Ellington in the Department of Molecular Biosciences, for example, enlisted Diaz early on in a project to explore leveraging machine learning to speed up the painstaking process of protein engineering. That project, known as MutCompute, was an early example of how AI can narrow the search space to tweak proteins and make them more stable, easier to produce or more accurate at hitting targets. The resulting AI model helped enable a breakthrough in plastic-eating enzymes.
Fast forward to today, and Diaz, now a research scientist in the Department of Computer Science at UT Austin, co-leads the Deep Proteins group, a team based in UT Austin’s Institute for Foundations of Machine Learning (IFML), which is funded by the National Science Foundation. Alongside the IFML director and Deep Proteins co-leader Adam Klivans, Diaz and other researchers collaborate with experimental scientists who produce and test AI-designed proteins in labs across UT as well as at partner institutions, including Houston Methodist Research Institute, the University of Minnesota and Cornell University.
“One of the things that makes UT special in this space is that we connect world-class AI researchers with world-class scientists in biochemistry to accelerate biotechnology development, all under one roof,” Klivans said. “Deep Proteins, which develops foundational protein AI tools with an eye towards real-world applications, was designed to be interdisciplinary from the start.”
Deep Proteins researchers draw on lessons from many disciplines, for example, to develop an AI technique called EvoRank that trains an AI model to learn from nature’s track record of protein evolution in order to predict evolutionarily plausible novel mutations.
Diaz realized that even if he didn’t have high quality mutational data from experiments in the lab, he could observe the diversity of proteins produced by nature.
“We wondered, can we learn the underlying rules nature used to produce this diversity?” he said. “Once these rules are learned by an AI model, we will be able to filter out mutations that kill proteins on the computer so we can focus on those that don’t in the lab, thus accelerating our ability to develop new protein-based biotechnologies.”
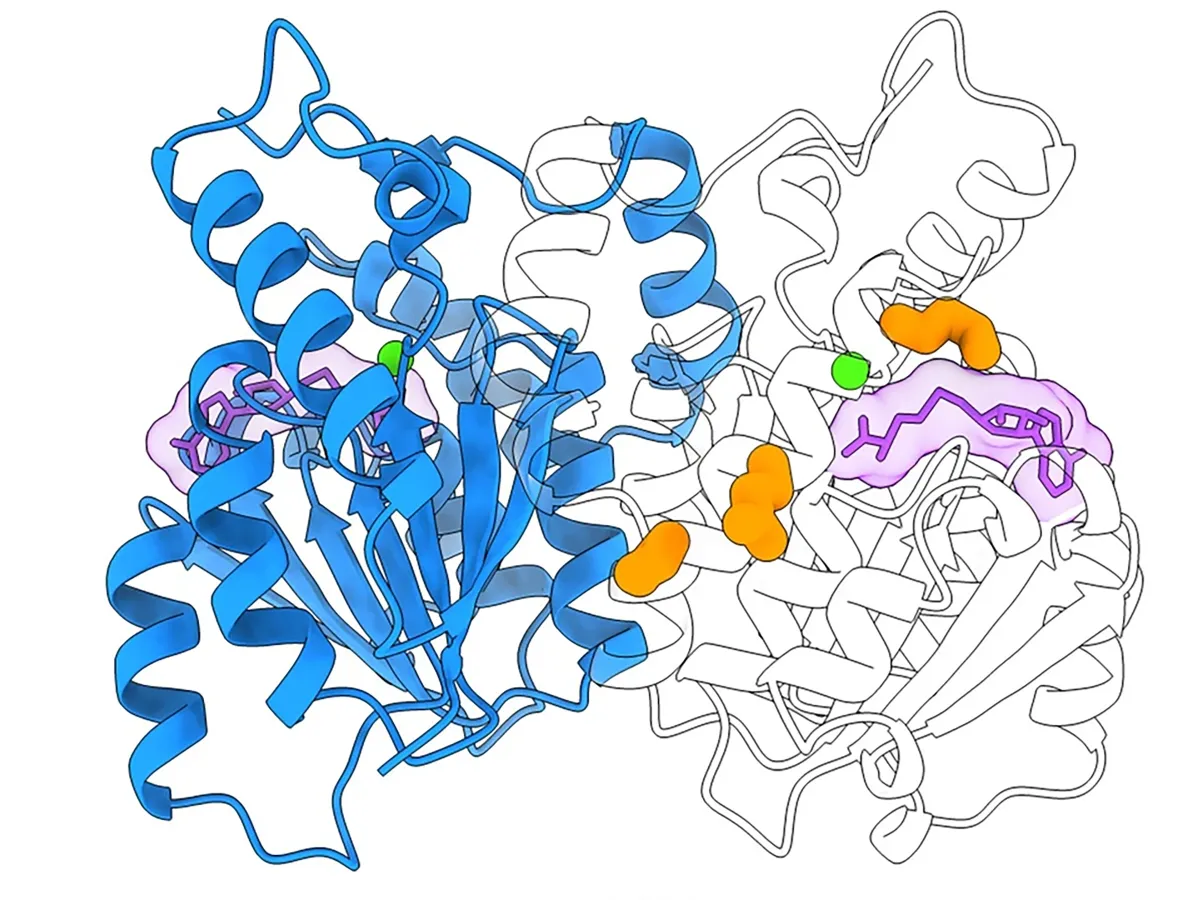
Protein Superpowers
To make commercially viable drugs, one of the superpowers researchers need to endow proteins with is the ability to be produced in high quantities, quickly and affordably. Working with professors Andrew Ellington, Jessie Zhang and others, Deep Proteins researchers used an AI model to do just that for an Alzheimer’s drug that is traditionally derived from daffodils, a costly and time-consuming process that is vulnerable to fickle growing conditions. They updated the model they had used earlier for the plastic-eating enzyme, extending it to the regions in proteins that interact with small molecules and vitamins.
“Proteins do not exist in isolation,” Diaz said. “They exist in a soup mixed with other proteins, RNA, DNA, vitamins, minerals, fats, sugars and other small molecules. Therefore, it’s critical that the AI is trained to understand not just protein chemistry but all of biochemistry.”
Guided by this updated AI model, MutComputeX, they E. coli bacteria to produce a precursor to the Alzheimer’s drug galantamine, allowing it to be grown in a lab more cheaply and reliably.
Boosting yield is also a key goal in a collaboration between the Deep Proteins group and research associate professor Everett Stone’s lab. That effort is seeking a treatment for a form of breast cancer that is highly dependent on a key metabolite called serine. Starting with a human enzyme that naturally breaks down serine, the researchers used their AI models to develop a more stable version that has seven times higher yield than the natural version. This version, which they’ve filed a patent for, is now in animal trials.
Another useful superpower is for a protein to bind more tightly to a target, a feature known as binding affinity. This generally means it will be more effective at a given task, such as when an antibody binds to a protein on the surface of a virus and prevents it from infecting cells. In a collaboration with Jimmy Gollihar at Houston Methodist Research Institute, Diaz used MutComputeX to dramatically increase the binding affinity of two antibodies against the virus that causes COVID-19.
Yet another protein superpower that’s especially useful for vaccines is stability, the knack for keeping its shape in the face of temperature or chemical changes experienced during the manufacturing and shipping process and once injected into your body.
Some viruses have proteins on their surfaces that allow them to infect cells and that are good at evading the immune system because they change shape. To generate the best immune response, vaccines based on these viral proteins have to lock them in the shape they typically take before infecting a cell. That was the key advance made by UT Austin professor of molecular biosciences Jason McLellan for the COVID-19 vaccines and RSV vaccines. McLellan’s lab is now working with the Deep Proteins group to explore how AI might help speed up the design process for future vaccines for other viruses, including as a part of a major new multi-million-dollar project led by La Jolla Institute for Immunology.
“We would normally spend a lot of time manually staring at structures, identifying amino acid substitutions, and maybe our hit rate wasn’t particularly high, like we’d have to try 100 substitutions to find 20 that worked,” said McLellan, one of the world’s leading vaccine innovators. “I think with machine learning, it’s going to have a higher hit rate eventually. Maybe we’ll get the same 20 substitutions, but only have to test 40.”
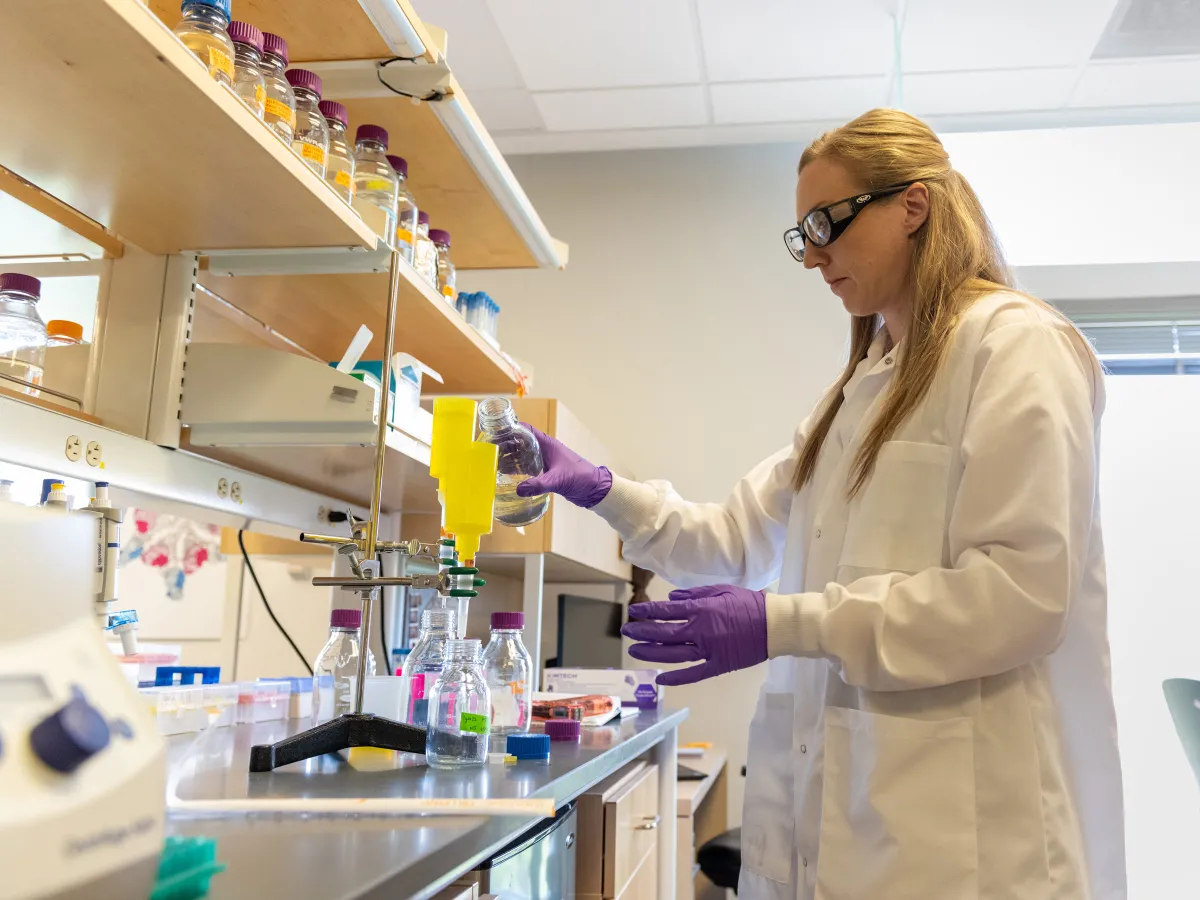
Predicting Stabilizing Mutations
Before developing the evolution-inspired technique EvoRank, Diaz, Klivans and Jeffrey Ouyang Zhang, a fourth-year computer science doctoral student, used their combined expertise to develop one particularly elegant algorithm, Mutate Everything. Starting from either AlphaFold or ESM, another AI model that predicts a protein’s structure based on its amino acid sequence, Mutate Everything can predict protein stability for single and higher-order mutations, with the aim of significantly accelerating research and development of protein-based biotechnologies. Building off their framework, Stability Oracle, which was recentlydescribed in a Nature Communications paper, the AI worked to identify and recommend single mutations for protein stabilization.
Mutate Everything will be retrained and updated as more protein experimental data becomes available, which makes the model important for applications in industries that rely on biotechnology, such as in the development of life-saving medications. The research team has made the algorithm publicly available to increase translation to industry use.
“Our computational tools can tell them how to stabilize a protein, which can save them a lot of time, headache and money,” Ouyang-Zhang said.
Still, AI researchers also must cope with having only limited experimental data on the properties of proteins to adequately train AI models to understand how proteins work and thus how to engineer them to work differently. That’s why newer efforts, like the Deep Proteins group’s work to learn from natural evolution via EvoRank, can complement the models that are based on experimental data.
Diaz is now working to launch a biotech startup company focused on inventing novel AI-inspired proteins to address obesity, diabetes and cancer.
“Nearly half of Americans are prediabetic or diabetic, and about as many will be diagnosed with cancer at some point in their life,” Diaz said. “Healthcare spending is currently outpacing GDP growth and currently nearly 20% of GDP. If we continue, these healthcare costs will decimate our country and put significant financial burdens on future generations. AI-engineered proteins can be the superheroes we need.”
Six years ago, when Diaz was getting started, the field of AI-guided protein engineering was brand new. Now the Deep Proteins team is among the most established and respected players in this space at a university anywhere. They also have a track record of AI-designed proteins to point to, with models that more traditional labs increasingly can benefit from.
“It’s hard to discover new things in established fields,” Diaz said. “With AI for protein engineering, there are lots of opportunities to do really cool, innovative work and that can change the world.”