Events
Workshop
-
Workshop on Seeking Low-dimensionality in Deep Neural Networks (SLowDNN)
Atlas Wang
Zoom
United States
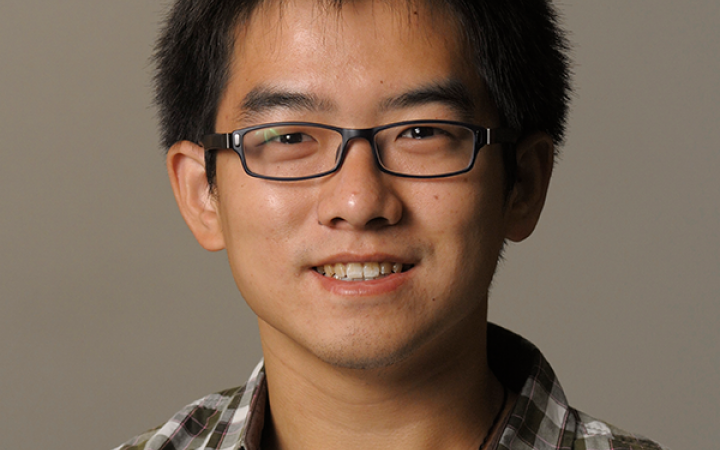
The resurgence of deep neural networks has led to revolutionary success across almost all the areas in engineering and science. However, despite recent endeavors, the underlying principles behind its success still remain a mystery. On the other hand, the connections between deep neural networks and low dimensional models emerge at multiple levels:
-
The structural connection between a deep neural network and a sparsifying algorithm has been well observed and acknowledged in the literature, which has also transformed the ways that we are solving inverse problems with intrinsic low-dimensionality.
-
Low-dimensional modeling has recently been shown as a commonly used testbed for understanding generalization, (implicit) regularization, expressivity, and robustness in over-parameterized deep learning models. For example, the learned representations of deep networks often possess certain benign low-dimensional structures, leading to better generalization and robustness.
-
Various theoretical and numerical evidence supports that enforcing certain isometry properties within the network often leads to improved performance for both training, generalization, and robustness.
-
Low-dimensional priors learned through deep networks demonstrated significantly improved performances over traditional methods in signal processing and machine learning.
Given these exciting, while less exploited connections, this two-day workshop aims to bring together experts in machine learning, applied mathematics, signal processing, and optimization, and share recent progress, and foster collaborations on mathematical foundations of deep learning. We would like to stimulate vibrate discussions towards bridging the gap between the theory and practice of deep learning by developing a more principled and unified mathematical framework based on the theory and methods for learning low-dimensional models in high-dimensional space.
Event Registration