Events
IFML Seminar
Recent Advances in Parallel Stochastic Convex Optimization
Kevin Tian, Assistant Professor, UT Austin
-The University of Texas at Austin
Gates Dell Complex (GDC 6.302)
United States
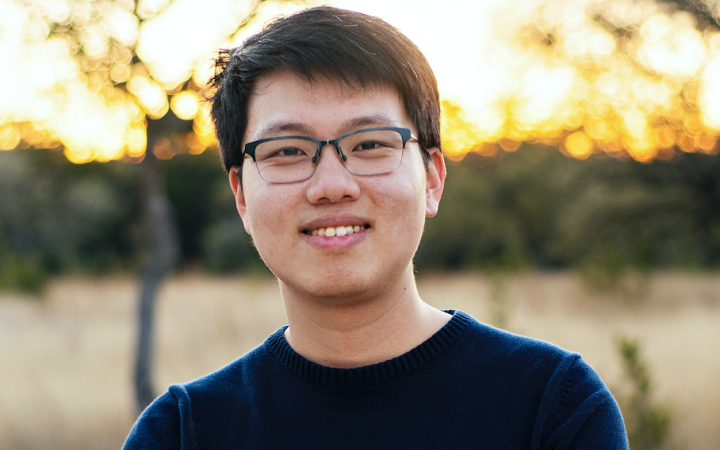
Abstract: The problem of parallel stochastic convex optimization (SCO) was first formalized by Nemirovski in ’94. In this problem, there is a convex function f: R^d —> R accessible only through a stochastic gradient estimator g unbiased for a subgradient of f everywhere, with bounded second moment. An algorithm is allowed to submit queries to g in parallel batches, and its complexity is measured through the query depth, computational depth, and total number of queries used by the algorithm. We overview two recent algorithms advancing the state-of-the-art for this problem, based on a new optimization primitive termed ball acceleration, combined with novel “locally-stable” gradient estimator constructions for the convolution of a Lipschitz function with a multivariate Gaussian density.
Based on joint works with Yair Carmon, Arun Jambulapati, Yujia Jin, Daogao Liu, Yin Tat Lee, and Aaron Sidford.
Speaker Bio: Kevin Tian is an Assistant Professor at UT Computer Science. Previously, Dr. Tian was a postdoctoral researcher at Microsoft Research Redmond from 2022-23, completed his Ph.D. in Computer Science at Stanford from 2016-2022 (advised by Aaron Sidford), and completed his B.S. in Mathematics and Computer Science at MIT from 2012-2015. Dr. Tian is interested in fundamental algorithmic problems in modern data science, often in the span of continuous optimization and high-dimensional statistics.
Event Registration