Events
IFML Seminar
A Margin-Theoretic Perspective on Feature Selection in Deep Networks
Matus Telgarsky, Assistant Professor University of Illinois Urbana-Champaign
-The University of Texas at Austin
GDC 6.302
United States
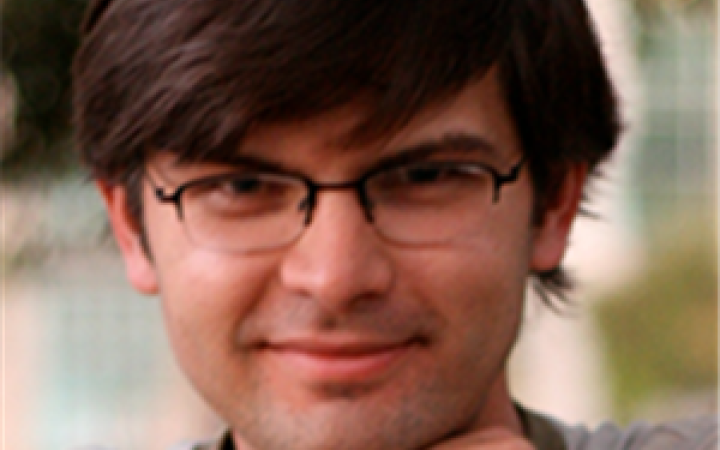
Abstract: This talk will first motivate and illustrate the use of margins as a way to interpret and analyze the behavior of deep network training, and then present a variety of results where this behavior can be analyzed, leading to learning guarantees with few samples, in particular in regimes where features move far from initialization. In further detail, the first part will illustrate a variety of behaviors of deep network training, and show how these correspond to margin maximization; notably, these behaviors can be counterintuitive, and contradict common beliefs regarding simplicity bias in deep networks. The second part will then present a variety of analyses where margins capture the motion of weights far from initialization; these analyses will be able to give the best sample and computational complexities for a variety of problems, and moreover the only general guarantees which are not forced to be near initialization.
Speaker Bio: Matus Telgarsky is an assistant professor at the University of Illinois, Urbana-Champaign, specializing in deep learning theory. He was fortunate to receive a PhD at UCSD under Sanjoy Dasgupta. Other highlights include: cofounding, in 2017, the Midwest ML Symposium (MMLS) with Po-Ling Loh; receiving a 2018 NSF CAREER award; and organizing two Simons Institute programs, one on deep learning theory (Summer 2019), and one on generalization (Fall 2024).