Events
IFML Seminar
IFML Seminar: 02/14/25 - Differentiable Weightless Neural Networks (DWNs)
Alan T. L. Bacellar, Ph.D student in the Electrical and Computer Engineering Department, UT Austin
-The University of Texas at Austin
Gates Dell Complex (GDC 6.302)
2317 Speedway
Austin, TX 78712
United States
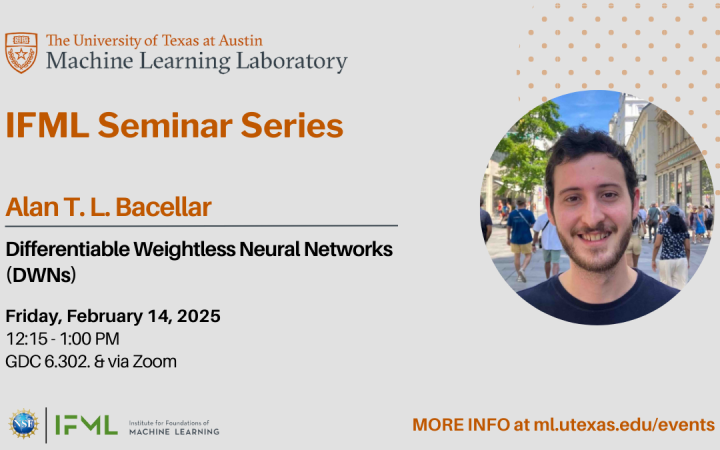
Abstract: Mainstream artificial neural network models, such as Deep Neural Networks (DNNs) are computation-heavy and energy-hungry. Weightless Neural Networks (WNNs) are natively built with RAM-based neurons and represent an entirely distinct type of neural network computing compared to DNNs. WNNs are extremely low-latency, low-energy, and suitable for efficient, accurate, edge inference. The WNN approach derives an implicit inspiration from the decoding process observed in the dendritic trees of biological neurons, making neurons based on Random Access Memories (RAMs) and/or Lookup Tables (LUTs) ready-to-deploy neuromorphic digital circuits.
In recent research [ICML2024], we introduced the Differentiable Weightless Neural Network (DWN), a model based on interconnected lookup tables. Training of DWNs is enabled by a novel Extended Finite Difference technique for approximate differentiation of binary values. We propose Learnable Mapping, Learnable Reduction, and Spectral Regularization to further improve the accuracy and efficiency of these models. We evaluated DWNs in three edge computing contexts: (1) an FPGA-based hardware accelerator, where they demonstrate superior latency, throughput, energy efficiency, and model area compared to state-of-the-art solutions, (2) a low-power microcontroller, where they achieve preferable accuracy to XGBoost while subject to stringent memory constraints, and (3) ultra-low-cost domain-specific chips, where they consistently outperform small models in both accuracy and projected hardware area. DWNs also compare favorably against leading approaches for tabular datasets, with higher average rank. Overall, our work positions DWNs as a pioneering solution for edge-compatible high-throughput neural networks. https://github.com/alanbacellar/DWN
Bio: Alan T. L. Bacellar is a Ph. D student in the Electrical and Computer Engineering Department at UT Austin, with Prof. Lizy K. John. He received his Bachelor’s Degree in Computation and Applied Mathematics at the Federal University of Rio de Janeiro, Brazil in 2023. He has published 12 papers on Weightless Neural Networks in conferences such as International Conference on Machine Learning (ICML), European Symposium on Artificial Neural Networks (ESANN), IFETC, MWSCAS, ACM Transactions on Architecture and Code Optimization (TACO), Neurocomputing, and IJCNN. He also won 1st Place in the International Joint Conferences on Neural Networks (IJCNN) 2021 Competition for Covid-19 Detection.
Event Registration