Events
IFML Seminar
IFML Seminar: 02/07/25 - Preference Optimization in Large Language Model Alignment: Personalization, Common Pitfalls and Beyond
Leqi Liu, assistant professor in use-inspired AI at the department of information, risk and operations management, UT Austin
-The University of Texas at Austin
Gates Dell Complex (GDC 6.302)
2317 Speedway
Austin, TX 78712
United States
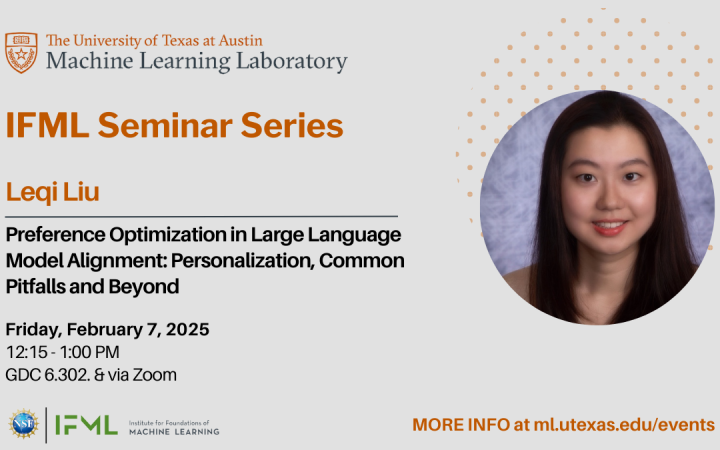
Abstract: Reinforcement Learning from Human Feedback (RLHF) has become the predominant method for aligning large language models (LLMs) to be more helpful and less harmful. In this talk, we address two core limitations of traditional RLHF. First, it assumes that all human preferences come from the same distribution, preventing fine-tuned LLMs from generating personalized content without explicit prompting. We introduce Personalized RLHF, an efficient framework that captures individual preferences through a lightweight user model, enabling LLMs to generate content that reflects diverse and potentially conflicting user preferences. Second, current RLHF methods often rely on optimizing against margin-based losses, which focus on the difference between preferred and dispreferred responses but fail to specify ideal LLM behavior on each type of the responses individually. This underspecification can lead to problematic training dynamics, increasing the probability of generating unsafe content or reducing the probability of generating ideal responses. We characterize when these problematic dynamics emerge and outline algorithms that can mitigate these issues. Finally, we will discuss future directions and potential new paradigms for improving LLM alignment.
Bio: Leqi Liu is an assistant professor in use-inspired AI at the department of information, risk and operations management at UT Austin. Her research focuses on (1) investigating the foundations of state-of-the-art machine intelligence, with a particular focus on generative AI systems; (2) designing principled algorithmic frameworks for human-centered machine learning that model human preferences and behaviors, integrating these models into machine learning pipelines for applications such as healthcare, recommender systems, and education; and (3) evaluating and auditing the societal impacts of large-scale AI systems, including large language models and recommender systems. She graduated from the Machine Learning Department at Carnegie Mellon University in 2023 where she was advised by Zachary Lipton, and spent a year at Princeton Language & Intelligence as a postdoc. She has also spent time at Apple and Google DeepMind London during her Ph.D., and was an Open Philanthropy AI Fellow.
Event Registration