Events
IFML Seminar
Foundation Model for Sequential Decision-Making
Furong Huang, Associate Professor, University of Maryland
-The University of Texas at Austin
Gates Dell Complex (GDC 6.302)
2317 Speedway
Austin, TX 78712
United States
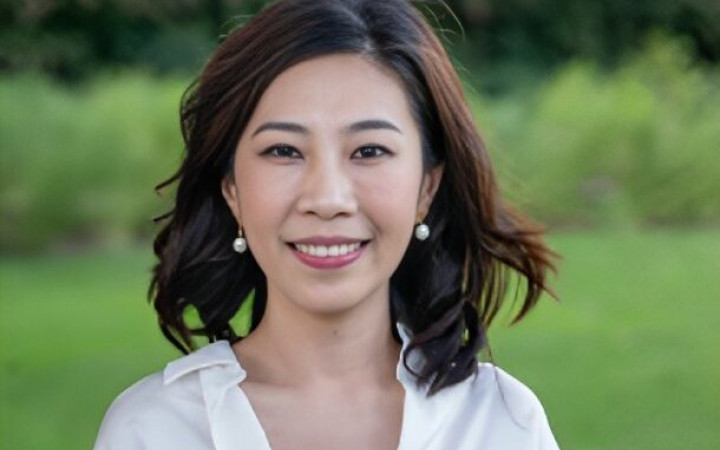
Abstract: Sequential decision-making (SDM) is crucial for adapting machine learning to dynamic real-world scenarios such as fluctuating markets or evolving healthcare, requiring models that can effectively navigate ongoing changes. Foundation models, akin to those in natural language processing like GPT and BERT, hold promise for similarly revolutionizing SDM by leveraging extensive datasets to manage the cascading effects of decisions in a constantly changing environment. This transition from pretraining to adaptation in foundation models for SDM unveils a triad of knowledge transfer avenues, each building on the foundational representation of states and actions. Our pivotal work, SMART, develops a general pretraining-finetuning pipeline for SDM, highlighted by the Self-supervised Multi-task pretrAining with contRol Transformer. Building on SMART, we introduce TACO, Temporal Action-driven Contrastive Learning, which sharpens state and action representations by optimizing the mutual information between current state-action sequences and subsequent state representations. To extend TACO's applicability across diverse tasks, we present Premier-TACO, a multitask approach to feature representation learning that enhances few-shot policy learning efficiency in SDM tasks.
Speaker Bio: Furong Huang is an Associate Professor of the Department of Computer Science at the University of Maryland. She received her Ph.D. in electrical engineering and computer science from UC Irvine in 2016, after which she spent one year as a postdoctoral researcher at Microsoft Research NYC. She works on statistical and trustworthy machine learning, foundation models and reinforcement learning, with specialization in domain adaptation, algorithmic robustness and fairness. With a focus on high-dimensional statistics and sequential decision-making, she develops efficient, robust, scalable, sustainable, ethical and responsible machine learning algorithms. She is recognized for her contributions with awards including best paper awards, the MIT Technology Review Innovators Under 35 Asia Pacific, the MLconf Industry Impact Research Award, the NSF CRII Award, the Microsoft Accelerate Foundation Models Research award, the Adobe Faculty Research Award, three JP Morgan Faculty Research Awards and Finalist of AI in Research - AI researcher of the year for Women in AI Awards North America.
Event Registration