Events
Ethics/Fairness in AI Seminar
Fairness for Generation with Uncertainty
Eric Price
-UT-Austin
United States
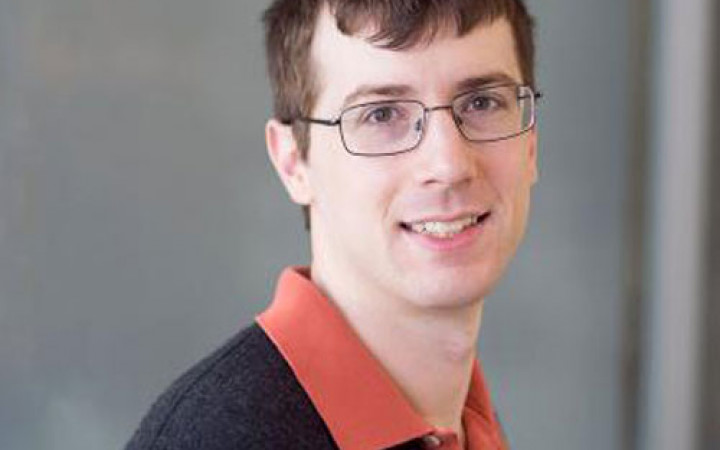
Traditional group fairness definitions are typically defined with
respect to a specified classification of people into protected groups,
despite many of these groupings being artificial. For instance, should
South and East Asians be viewed as a single group or separate groups?
Should we consider one race as a whole or further split groupings by
gender? Choosing which groups are valid and who belongs in them is an
impossible dilemma and being “fair” with respect to Asians may require
being “unfair” with respect to South Asians. Ideally, one would like
definitions that allow algorithms to be fair for any grouping.
We consider fairness in the context of generative procedures, such as
image super-resolution or inpainting. We show how maximum-accuracy
generators can lead to underrepresentation of minority groups. We
then introduce a new fairness definition, proportional representation,
and compare it to existing approaches. In addition to an intuitive
justification in terms of representation, proportional representation
has a key advantage over other definitions: it is achievable
obliviously to the grouping. The algorithm that achieves this,
posterior sampling, is also accurate in every metric. Our experiments
validate our theoretical results and achieve fair state-of-the-art
image reconstruction.
Based on joint work with Alex Dimakis, Jessica Hoffman, Ajil Jalal,
and Sushrut Karmalkar.