Events
Evaluating AI-based MR image reconstruction models: lessons from the fastMRI project
Matthew Muckley
-Zoom
United States
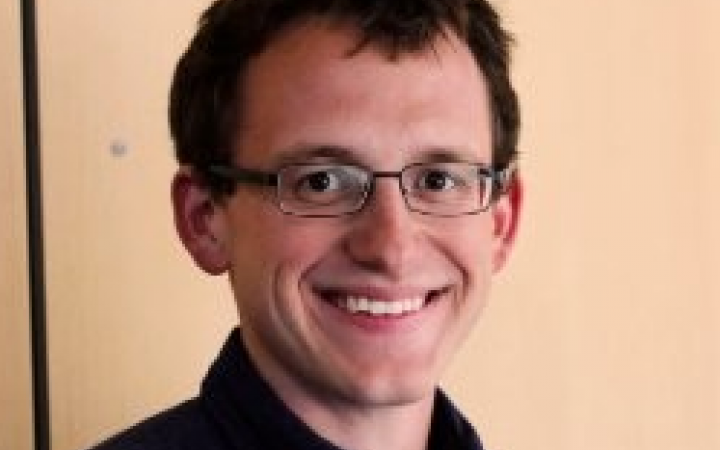
Abstract: fastMRI is a collaboration project between Facebook AI Research and the NYU School of Medicine with the goal of reducing the time to acquire an MRI scan. As part of the collaboration, the team released the largest public dataset of raw MRI k-space measurements with an associated website, leaderboards, and GitHub repository. Use of AI models for medical image reconstruction carries the key attribute that the images are evaluated for pathology by human radiologists. I will discuss two outcomes of the project that included radiologists in the loop of the evaluation process. The first outcome is the development of a reconstruction model that achieved clinical interchangeability for knee scans while reducing the scan time to less than 5 minutes. The second outcome is the successful launch and conclusion of two open competitions for MRI reconstructions based on the dataset. I will conclude with a summary of ongoing work for estimating ground-truth error metrics with only undersampled data.
Bio: Matthew Muckley is a research engineer at Facebook AI Research. He completed his PhD on MRI acceleration methods in 2016 at the University of Michigan. Following his PhD, he spent 3.5 years at the NYU School of Medicine working on X-ray CT and MRI reconstruction methods. Since February of 2020, he has been at FAIR, where his work has continued to focus on medical imaging as well as maintaining the fastMRI and NeuralCompression open-source code repositories. His current research interests are on the use of neural networks for data compression and inverse problems.