Events
IFML Seminar
Engineering for Efficiency and Equity in Resident Crowdsourcing
Nikhil Garg, Assistant Professor at Cornell Tech
-The University of Texas at Austin
Gates-Dell Complex (GDC 6.302)
United States
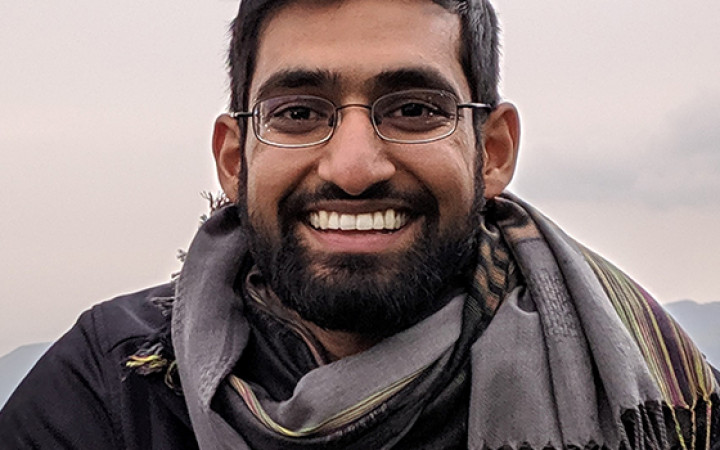
Abstract: Modern city governance relies heavily on crowdsourcing to identify problems such as downed trees and power-lines. Two major concerns are that (1) residents do not report problems at the same rates and (2) agencies differentially respond to reports, leading to an inefficient and inequitable allocation of government resources. However, measuring and correcting such under-reporting and differential responses are challenging statistical tasks: ground truth incident and risk distributions may differ by area, and, almost by definition, we do not observe incidents that are not reported. We develop statistical methods to overcoming such missing and censored data challenges, leveraging domain-specific information such as duplicate reports and spatial correlation. We apply our methods to over 100,000 resident reports made to the New York City Department of Parks and Recreation, finding that there are substantial spatial and socio-economic disparities, even after controlling for incident characteristics. Finally, I'll overview our work in translating the data science/machine learning insights to practice, to influence agency response to reports.
Speaker Bio: Nikhil Garg is an Assistant Professor at Cornell Tech. Garg’s research is at the intersection of computer science, economics, and operations—on the application of algorithms, data science, and mechanism design to the study of democracy, markets, and societal systems at large. His research interests include surge pricing, rating systems, how to vote on budgets, the role of testing in college admissions, stereotypes in word embeddings, and polarization on Twitter. He received his Ph.D. from Stanford in 2020, where he was part of the Society and Algorithms Lab and Stanford Crowdsourced Democracy Team. He also received a B.S. and B.A. degrees from UT Austin in 2015. He has spent time at Uber, NASA, Microsoft, the Texas Senate, and IEEE's policy arm, and most recently was the principal data scientist at PredictWise—which provides election analytics for political campaigns—and is currently completing a postdoc at the University of California, Berkeley in the Department of Electrical Engineering and Computer Science.
Event Registration