Events
ECE Imaging Seminar: Combining Machine Learning Tools with Physics for Applications in Accelerated MRI
Yamin Arefeen, PhD Candidate, MIT
-The University of Texas at Austin EER 3.640
United States
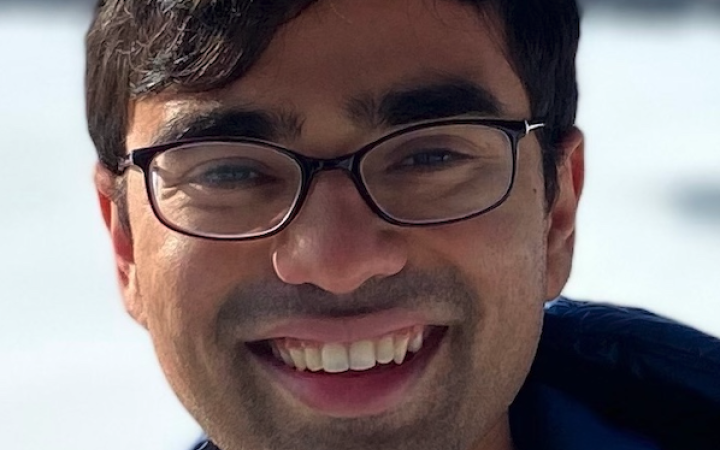
Abstract: Magnetic Resonance Imaging (MRI) enables non-invasive measurement of anatomy with a wide range of image contrast, but incurs significant costs to patients due to expensive equipment and slow imaging times. Over the last few years, a number of data-driven methods have been proposed to reduce acquisition times, but these techniques often require large training databases that may be infeasible or expensive to acquire in some MRI settings.
This talk presents two applications that combine data-driven tools with MRI physics to enable accelerated acquisitions without measured training datasets. (1) First, we discuss Latent Signal Models, a technique that learns compact latent representations of signal from simulated data for time-resolved MRI. We show that our proposed approach improves reconstructions in comparison to linear models through reduced degrees of freedom and serves as an efficient proxy for optimizing with the Bloch Equations. (2) Then, we cover SPARK, a scan-specific machine learning technique for accelerated structural MRI that corrects reconstruction artifacts in k-space given some input reconstruction. SPARK is trained on just the acquired dataset, requires less calibration data than previous techniques, and synergizes with a wide range of physics based reconstruction algorithms.
Speaker Bio: Yamin Arefeen is a PhD candidate in Electrical Engineering and Computer Science at the Massachussetts Institute of Technology (MIT) under the supervision of Elfar Adalsteinsson and Berkin Bilgic. He received his S.M. degree from MIT and B.S. degree from Rice University in Electrical and Computer Engineering. He is interested in combining computational techniques with imaging physics to improve medical imaging, with his PhD work focusing on applications in MRI.
The seminar is hosted by the UT Computational Sensing and Imaging Lab.