Events
Use-Inspired Research Seminar
Clustering Mixtures with Almost Optimal Separation in Polynomial Time
Allen Liu. Ph.D. student at Massachusetts Institute of Technology
-Microsoft Research
United States
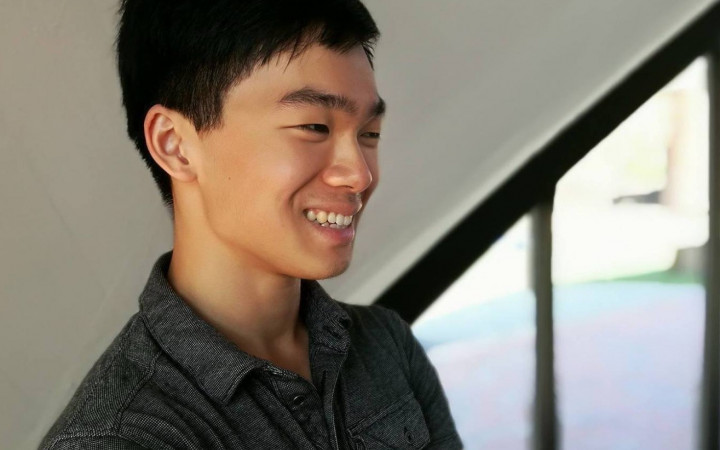
Clustering Mixtures with Almost Optimal Separation in Polynomial Time
Jerry Li, Allen Liu
Abstract: We consider the problem of clustering mixtures of mean-separated Gaussians in high dimensions. We are given samples from a mixture of k identity covariance Gaussians, so that the minimum pairwise distance between any two pairs of means is at least ∆, for some parameter ∆ > 0, and the goal is to recover the ground truth clustering of these samples. It is folklore that separation ∆ = Θ(log^{1/2} k) is both necessary and sufficient to recover a good clustering, at least information theoretically. However, the estimators which achieve this guarantee are inefficient. We give the first algorithm which runs in polynomial time, and which almost matches this guarantee. More precisely, we give an algorithm which takes polynomially many samples and time, and which can successfully recover a good clustering, so long as the separation is ∆ = Ω(log^{1/2+c} k), for any c > 0. Previously, polynomial time algorithms were only known for this problem when the separation was polynomial in k, and all algorithms which could tolerate poly(log k) separation required quasi-polynomial time. We also extend our result to mixtures of translations of a distribution which satisfies the Poincaré inequality, under additional mild assumptions. Our main technical tool, which we believe is of independent interest, is a novel way to implicitly represent and estimate high degree moments of a distribution, which allows us to extract important information about high-degree moments without ever writing down the full moment tensors explicitly.
Speaker Bio: Allen Liu is currently a first-year graduate student in EECS at MIT. Part of this work was completed during an internship at Microsoft Research with Jerry Li. Liu earned his undergraduate degree in mathematics at MIT. He's interested in algorithms and learning theory, particularly developing algorithms for machine learning with provable guarantees. His work is supported by an NSF Graduate Research Fellowship and a Hertz Fellowship.