Events
Foundational Research Seminar
Bootstrapping the Error of Oja's algorithm
Purnamrita Sarkar
-UT-Austin
United States
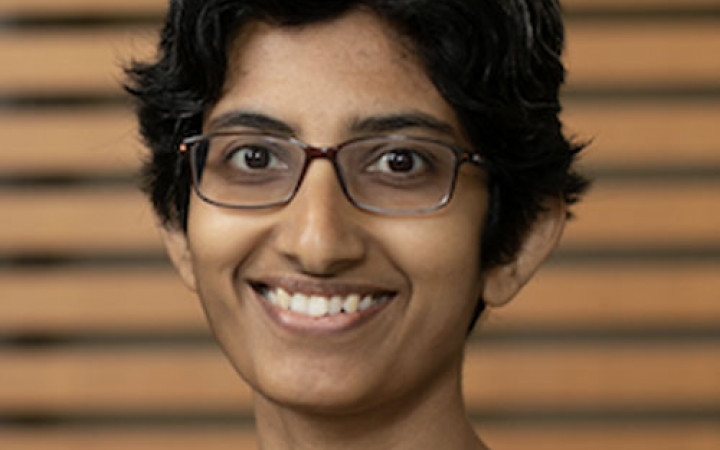
We consider the problem of quantifying uncertainty for the estimation error of the leading eigenvector from Oja's algorithm for streaming Principal Component Analysis, where the data are generated IID from some unknown distribution. By combining classical tools from the U-statistics literature with recent results on high-dimensional central limit theorems of quadratic forms of random vectors and concentration of matrix products, we establish a Gaussian approximation result for the sin squared distance between the population eigenvector and the output of Oja’s algorithm. Since estimating the covariance matrix associated with the approximating distribution requires knowledge of unknown model parameters, we propose a multiplier bootstrap algorithm that may be updated in an online manner. We establish conditions under which the bootstrap distribution is close to the corresponding sampling distribution with high probability, thereby establishing the bootstrap as a consistent inferential method in an appropriate asymptotic regime. This is joint work with Robert Lunde and Rachel Ward.