Events
IFML Seminar
Activation Function Design for Deep Networks: Linearity and Effective Initialisation
Jared Tanner, PhD, Professor of Mathematics of Information, University of Oxford
-The University of Texas at Austin
Gates Dell Complex (GDC 6.302)
United States
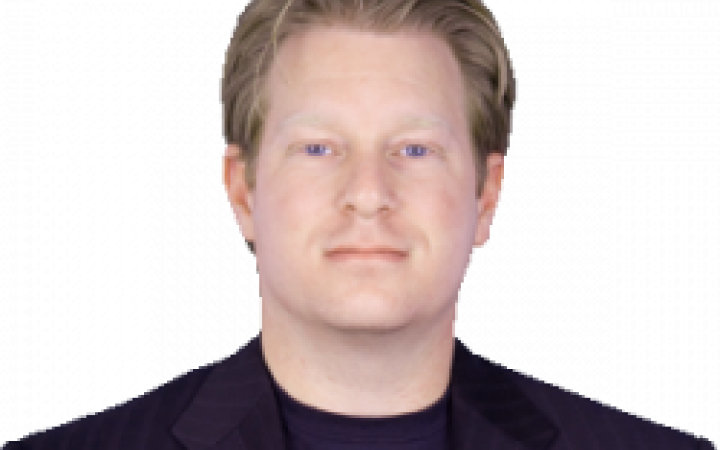
Abstract: The activation function deployed in a deep neural network has great influence on the performance of the network at initialisation, which in turn has implications for training. In this paper we study how to avoid two problems at initialisation identified in prior works: rapid convergence of pairwise input correlations, and vanishing and exploding gradients. We prove that both these problems can be avoided by choosing an activation function possessing a sufficiently large linear region around the origin, relative to the bias variance σb of the network’s random initialisation. We demonstrate empirically that using such activation functions leads to tangible benefits in practice, both in terms test and training accuracy as well as training time. Furthermore, we observe that the shape of the nonlinear activation outside the linear region appears to have a relatively limited impact on training. Finally, our results also allow us to train networks in a new hyperparameter regime, with a much larger bias variance than has previously been possible. This work is joint with Michael Murray (UCLA) and Vinayak Abrol (IIIT Delhi).
Bio: Jared Tanner has been a Professor of the Mathematics of Information at the University of Oxford since 2012. Prior to his current position, he was a lecturer, reader, and professor at the University of Edinburgh from 2007-2012 and an assistant professor at the University of Utah in 2006-2007. He received his doctorate in applied maths from UCLA in 2002, held postdoctoral positions at UC Davis (2002-2004) and Stanford University (2004-2006). He has contributed algorithms, theory, and applications of compressed sensing as well as more recently to deep learning.
Event Registration